
The Celeste graphical model. Shaded vertices represent observed random variables. Empty vertices represent latent ran- dom variables. Black dots represent constants. Constants denoted by uppercase Greek characters are fixed and denote parameters of prior distributions. The remaining constants and all latent random variables are inferred.
Berkeley Lab-based research collaboration of astrophysicists, statisticians and computer scientists is looking to shake things up with Celeste, a new statistical analysis model designed to enhance one of modern astronomy’s most time-tested tools: sky surveys. A central component of an astronomer’s daily activities, surveys are used to map and catalog regions of the sky, fuel statistical studies of large numbers of objects and enable interesting or rare objects to be studied in greater detail. But the ways in which image datasets from these surveys are analyzed today remains stuck in the Dark Ages.
Surprisingly, the first electronic survey -the Sloan Digital Sky Survey (SDSS)- only began capturing data in 1998. And while today there are multiple surveys and high-resolution instrumentation operating 24/7 worldwide and collecting hundreds of terabytes of image data annually, the ability of scientists from multiple facilities to easily access and share this data remains elusive. Also practices originating a hundred years ago or more continue to proliferate in astronomy- from the habit of approaching each survey image analysis as though it were the first time they’ve looked at the sky to antiquated terminology such as “magnitude system” and “sexagesimal”.

DeCAM/DeCALs image of galaxies, observed by the Blanco Telescope. The Legacy Survey is producing an inference model catalog of the sky from a set of optical and infrared imaging data, comprising 14,000 deg² of extragalactic sky visible from the northern hemisphere in three optical bands and four infrared bands. Image: Dark Energy Sky Survey
“The way we deal with data analysis in astronomy is through ‘data reduction,'” he said. “You take an image, apply a detection algorithm to it, take some measurements and then make a catalog of the objects in that image. Then you take another image of the same part of the sky and you say ‘Oh, let me pretend I don’t know what’s going on here, so I’ll start by identifying objects, taking measurements of those objects and then make a catalog of those objects.’ And this is done independently for each image. So you keep stepping further and further down into these data reduction catalogs and never going back to the original image.”
These challenges prompted Schlegel to team up with Berkeley Lab’s MANTISSA (Massive Acceleration of New Technologies in Science with Scalable Algorithms) project, led by Prabhat (from NERSC).
The team spent the past year developing Celeste, a hierarchical model designed to catalog stars, galaxies and other light sources in the universe visible through the next generation of telescopes. It will also enable astronomers to identify promising galaxies for spectrograph targeting, define galaxies they may want to explore further and help them better understand Dark Energy and the geometry of the universe, he added. Celeste also will reduce the time and effort that astronomers currently spend working with image data, Schlegel emphasized.
The model is fashioned on a code called the Tractor…” instead of running fairly simple recipes on pixel values, we create a full, descriptive model that we can compare to actual images and then adjust the model so that its claims of what a particular star actually looks like match the observations. It makes more explicit statements about what objects exist and predictions of what those objects will look like in the data.”
The Celeste project implements statistical inference to build a fully generative model to mathematically locate and characterize light sources in the sky. So far the group has used Celeste to analyze pieces of SDSS images, whole SDSS images and sets of SDSS images on NERSC’s Edison supercomputer. These initial runs have helped them refine and improve the model and validate its ability to exceed the performance of current state-of-the-art methods for locating celestial bodies and measuring their colors.
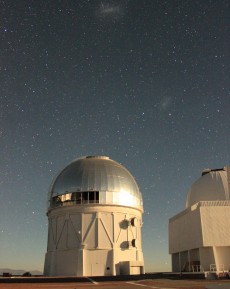
The VÃctor M. Blanco Telescope at the Cerro Tololo Inter-American Observatory in Chile, where the Dark Energy Camera is being used to collect image data for the DECam Legacy Survey. The glint off the dome is moonlight; the small and large Magellanic clouds can be seen in the background. Image: Dustin Lang, University of Toronto
“The ultimate goal is to take all of the photometric data generated up to now and that is going to be generated on an ongoing basis and run a single job and keep running it over time and continually refine this comprehensive catalog,” he said..
The first major milestone will be to run an analysis of the entire SDSS dataset all at once at NERSC. The researchers will then begin adding other datasets and begin building the catalog–which, like the SDSS data, will likely be housed on a science gateway at NERSC. In all, the Celeste team expects the catalog to collect and process some 500 terabytes of data, or about 1 trillion pixels.
The next iteration of Celeste will include quasars, which have a distinct spectral signature that makes them more difficult to distinguish from other light sources. The modeling of quasars is important to improving our understanding of the early universe, but it presents a big challenge: the most important objects are those that are far away, but distant objects are the ones for which we have the weakest signal. http://newscenter.lbl.gov/2015/09/09/celeste-a-new-model-for-cataloging-the-universe/
Recent Comments