
Large language models (LLMs), the most renowned of which is ChatGPT, have become increasingly better at processing and generating human language over the past few years. The extent to which these models emulate the neural processes supporting language processing by the human brain, however, has yet to be fully elucidated.
Researchers at Columbia University and Feinstein Institutes for Medical Research Northwell Health recently carried out a study investigating the similarities between LLM representations on neural responses. Their findings, published in Nature Machine Intelligence, suggest that as LLMs become more advanced, they do not only perform better, but they also become more brain-like.
“Our original inspiration for this paper came from the recent explosion in the landscape of LLMs and neuro-AI research,” Gavin Mischler, first author of the paper, told Tech Xplore.
“A few papers over the past few years showed that the word embeddings from GPT-2 displayed some similarity with the word responses recorded from the human brain, but in the fast-paced domain of AI, GPT-2 is now considered old and not very powerful.
“Ever since ChatGPT was released, there have been so many other powerful models that have come out, but there hasn’t been much research on whether these newer, bigger, better models still display those same brain similarities.”
The main objective of the recent study by Mischler and his colleagues was to determine whether the latest LLMs also exhibit similarities with the human brain. This could improve the understanding of both artificial intelligence (AI) and the brain, particularly in terms of how they analyze and produce language.
The researchers examined 12 different open-source models developed over the past few years, which have almost identical architectures and a similar number of parameters. Concurrently, they also recorded neural responses in the brains of neurosurgical patients as they listened to speech, using electrodes that were implanted in their brains as part of their treatment.
“We also gave the text of the same speech to the LLMs and extracted their embeddings, which are essentially the internal representations that the different layers of an LLM use to encode and process the text,” explained Mischler.
“To estimate the similarity between these models and the brain, we tried to predict the recorded neural responses to words from the word embeddings. The ability to predict the brain responses from the word embeddings gives us a sense of how similar the two are.”
After collecting their data, the researchers used computational tools to determine the extent to which LLMs and the brain were aligned. They specifically looked at what layers of each LLM showed the greatest correspondence with brain regions involved in language processing, in which neural responses to speech are known to gradually “build up” language representations by examining acoustic, phonetic and eventually more abstract components of speech.
“First, we found that as LLMs get more powerful (for example, as they get better at answering questions like ChatGPT), their embeddings become more similar to the brain’s neural responses to language,” said Mischler.
“More surprisingly, as LLM performance increases, their alignment with the brain’s hierarchy also increases. This means that the amount and type of information extracted over successive brain regions during language processing aligns better with the information extracted by successive layers of the highest-performing LLMs than it does with low-performing LLMs.”
The results gathered by this team of researchers suggest that the best performing LLMs mirror brain responses associated with language processing more closely. Moreover, their better performance appears to be due to the greater efficiency of their earlier layers.
“These findings have various implications, one of which is that the modern approach to LLM architectures and training is leading these models toward the same principles employed by the human brain, which is incredibly specialized in language processing,” said Mischler.
“Whether this is because there are some fundamental principles that underly the most efficient way to understand language, or simply by chance, it appears that both natural and artificial systems are converging toward a similar method for language processing.”
The recent work by Mischler and his colleagues could pave the way for further studies comparing LLM representations and neural responses associated with language processing. Collectively, these research efforts could inform the development of future LLMs, ensuring that they better align with human mental processes.
“I think the brain is so interesting because we still don’t totally understand how it does what it does, and its language processing ability is uniquely human,” added Mischler. “At the same time, LLMs are in some ways still a black box despite being capable of some amazing things, so we want to try to use LLMs to understand the brain and vice versa.
“We now have new hypotheses about the importance of early layers in high-performing LLMs, and by extrapolating the trend of better LLMs showing better brain-correspondence, perhaps these results can provide some potential ways to make LLMs more powerful by explicitly making them more brain-like.” https://techxplore.com/news/2024-12-llms-brain-advance.html
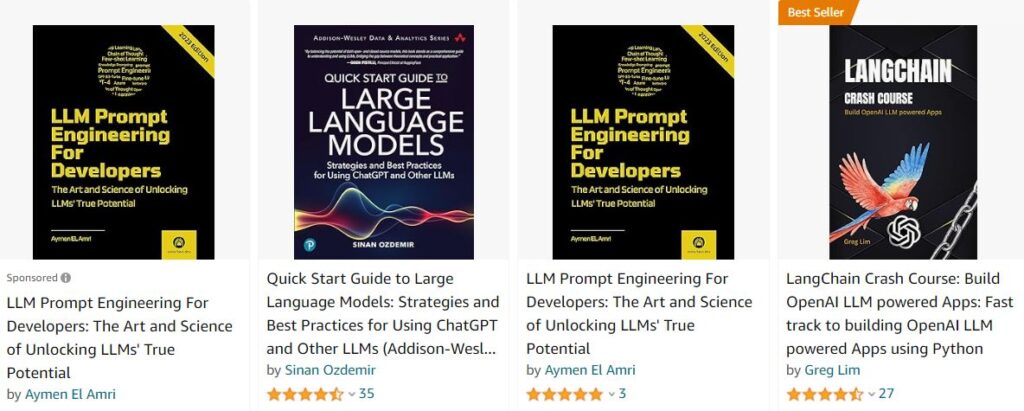
Recent Comments