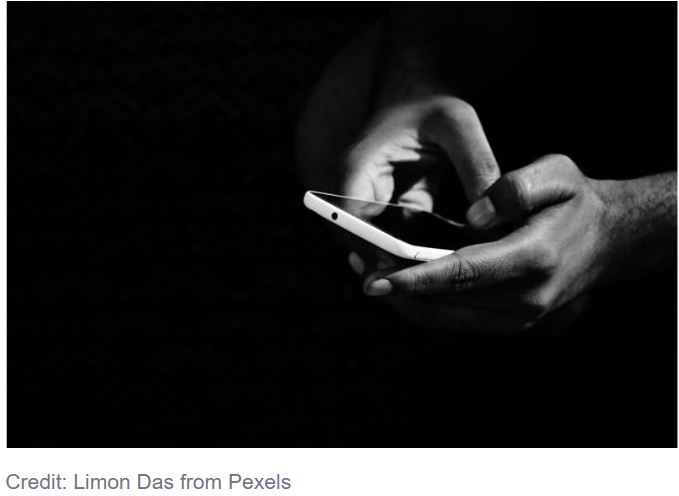
Large language models (LLMs) are increasingly automating tasks like translation, text classification and customer service. But tapping into an LLM’s power typically requires users to send their requests to a centralized server—a process that’s expensive, energy-intensive and often slow.
Now, researchers have introduced a technique for compressing an LLM’s reams of data, which could increase privacy, save energy and lower costs. Their findings are published on the arXiv preprint server.
The new algorithm, developed by engineers at Princeton and Stanford Engineering, works by trimming redundancies and reducing the precision of an LLM’s layers of information...
Read More
Recent Comments