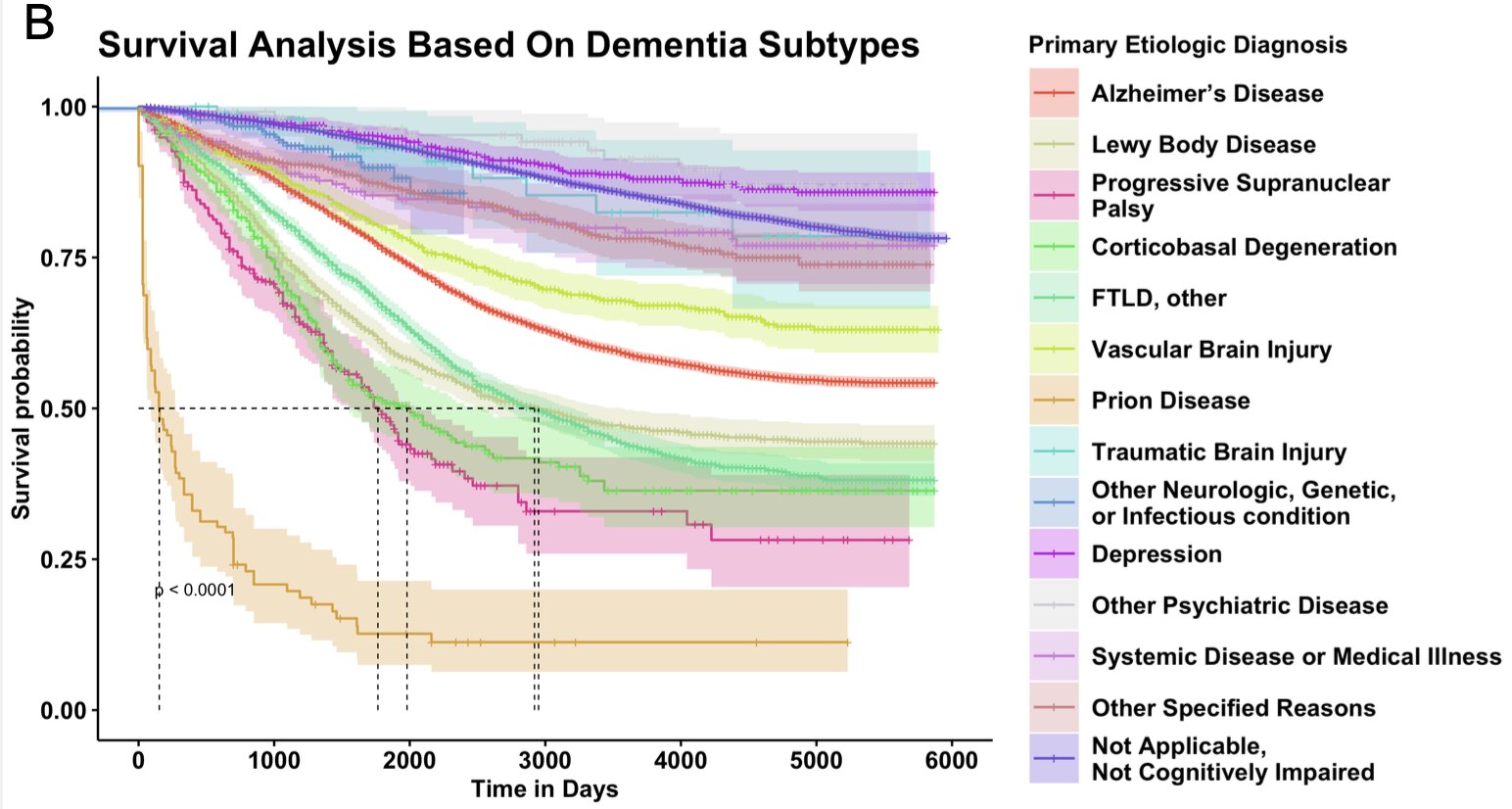
Researchers at the Icahn School of Medicine at Mount Sinai and others have harnessed the power of machine learning to identify key predictors of mortality in dementia patients.
The study, published in the February 28 online issue of Communications Medicine, addresses critical challenges in dementia care by pinpointing patients at high risk of near-term death and uncovers the factors that drive this risk.
Unlike previous studies that focused on diagnosing dementia, this research delves into predicting patient prognosis, shedding light on mortality risks and contributing factors in various kinds of dementia.
Dementia has emerged as a major cause of death in societies with increasin...
Read More
Recent Comments