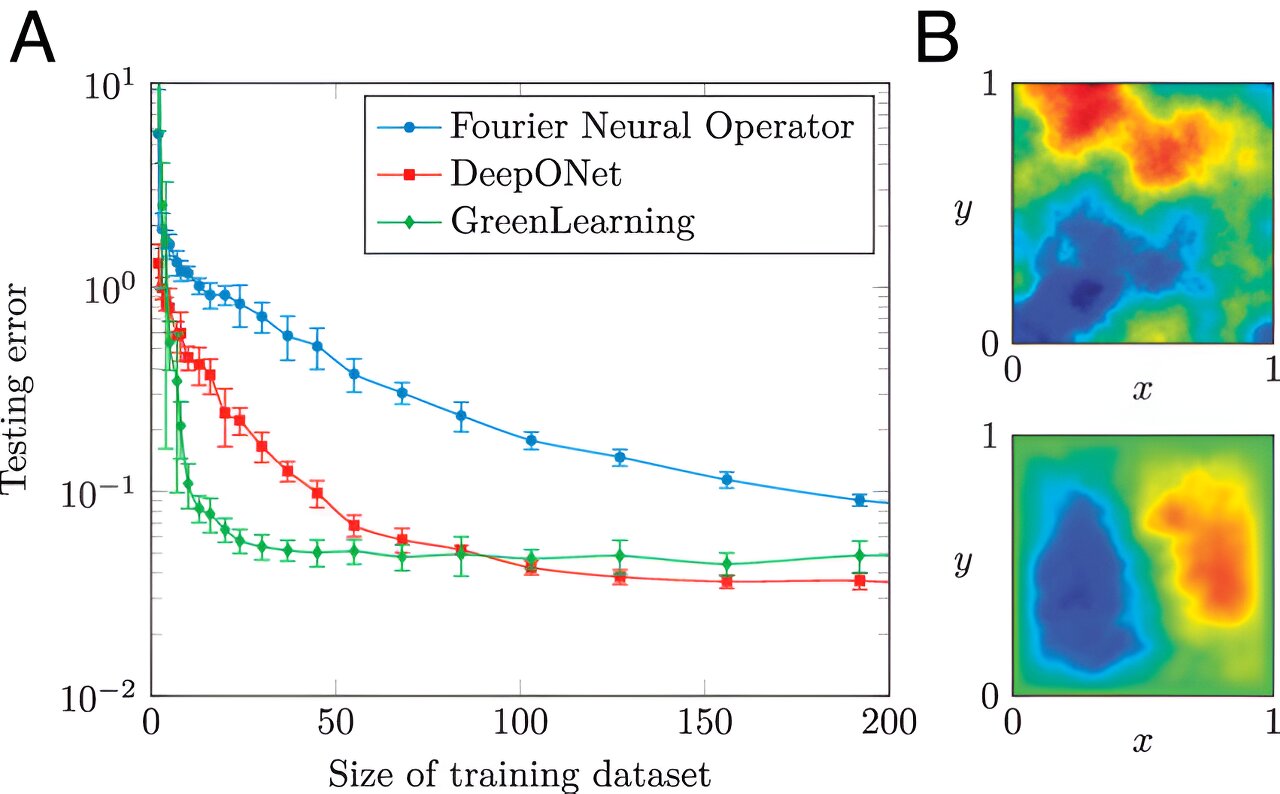
Researchers have determined how to build reliable machine learning models that can understand complex equations in real-world situations while using far less training data than is normally expected.
The researchers, from the University of Cambridge and Cornell University, found that for partial differential equations—a class of physics equations that describe how things in the natural world evolve in space and time—machine learning models can produce reliable results even when they are provided with limited data.
Their results, reported in the Proceedings of the National Academy of Sciences, could be useful for constructing more t...
Read More
Recent Comments